Embracing Continuous Machine Learning: A Paradigm for Modern AI Development
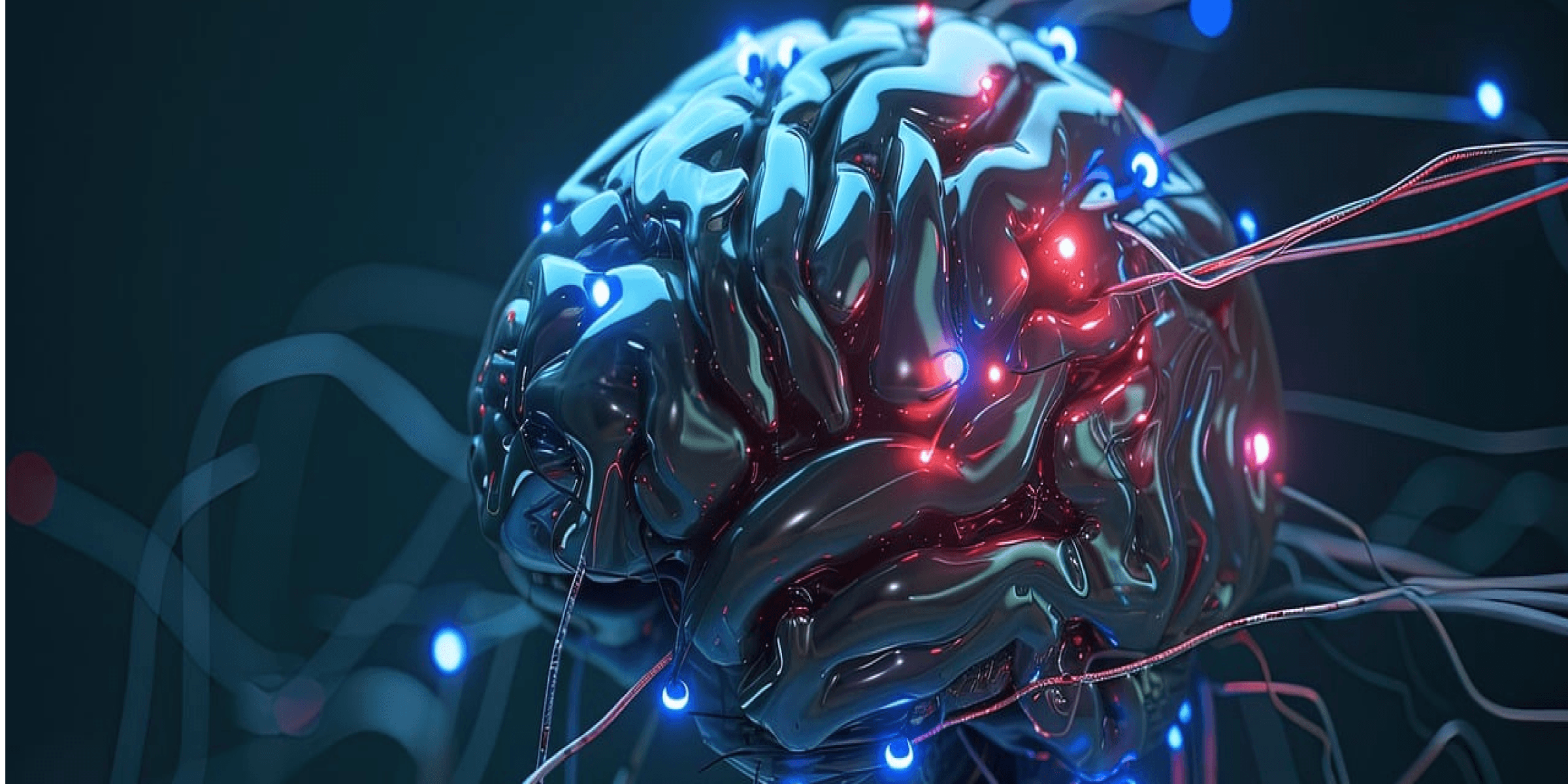
In the rapidly evolving world of Artificial Intelligence (AI) and Machine Learning (ML), staying ahead of the curve is crucial. One of the most transformative advancements in this field is Continuous Machine Learning (CML), a methodology that emphasizes the ongoing improvement and adaptation of machine learning models. This blog delves into the concept of Continuous Machine Learning, exploring its significance, applications, and best practices.
What is Continuous Machine Learning?
Continuous Machine Learning is an iterative process that involves the regular updating and refining of machine learning models based on new data and feedback. Unlike traditional ML models that are trained once and deployed, CML emphasizes the importance of maintaining and improving models throughout their lifecycle. This approach ensures that models remain accurate, relevant, and resilient to changes in the data environment.
Why is Continuous Machine Learning Important?
1. Adaptability: Data is constantly evolving. Continuous Machine Learning allows models to adapt to new patterns, trends, and anomalies, ensuring they stay relevant over time.
2. Accuracy: Regular updates and refinements help in correcting drifts and biases, maintaining high levels of accuracy in predictions.
3. Efficiency: Automated processes in CML reduce the time and effort required for model maintenance, allowing data scientists to focus on more strategic tasks.
4. Scalability: Continuous learning frameworks can handle growing volumes of data and increasingly complex models, making them scalable solutions for businesses.
Key Components of Continuous Machine Learning
1. Automated Data Pipelines: Setting up automated data ingestion pipelines ensures that models receive fresh data continuously. This involves data cleaning, transformation, and validation processes to maintain data quality.
2. Monitoring and Logging: Continuous monitoring of model performance is crucial. Logging key metrics like accuracy, precision, recall, and response times helps in identifying when a model needs retraining or adjustment.
3. Automated Retraining: Implementing automated retraining schedules or triggers based on performance metrics ensures that models are updated regularly without manual intervention.
4. Version Control: Using version control for models and data allows for tracking changes, rolling back to previous versions if necessary, and maintaining a history of model evolution.
5. Feedback Loops: Incorporating feedback from users or systems into the model training process can enhance model performance and ensure it aligns with real-world requirements.
Applications of Continuous Machine Learning
1. E-commerce: Personalized recommendations and dynamic pricing models benefit significantly from continuous learning, adapting to changing customer preferences and market conditions.
2. Finance: Fraud detection systems need to adapt to new fraud patterns and techniques. Continuous Machine Learning ensures these systems remain effective.
3. Healthcare: Diagnostic models and patient monitoring systems must constantly update to incorporate new medical research and patient data.
4. Manufacturing: Predictive maintenance models that continuously learn from new sensor data can more accurately predict equipment failures and reduce downtime.
Best Practices for Implementing Continuous Machine Learning
1. Start Small: Begin with a pilot project to establish the feasibility and benefits of CML within your organization. This helps in fine-tuning processes before scaling up.
2. Ensure Data Quality: High-quality data is the backbone of effective CML. Invest in robust data governance and cleaning processes.
3. Leverage Automation Tools: Utilize existing ML Ops tools and platforms that offer automation features for data pipelines, monitoring, and retraining.
4. Cross-functional Teams: Collaborate across data science, IT, and business teams to align model development with organizational goals and ensure smooth implementation.
5. Continuous Evaluation: Regularly evaluate the performance and impact of your models, incorporating user feedback and business metrics to guide improvements.
Future of Continuous Machine Learning
As AI technology advances, the integration of Continuous Machine Learning will become increasingly seamless and sophisticated. Future developments may include more advanced algorithms for automated model tuning, enhanced tools for real-time data processing, and more intuitive interfaces for monitoring and managing ML models. Additionally, the rise of edge computing will enable continuous learning at the device level, opening new possibilities for real-time, on-the-fly model updates in various applications.
Conclusion
Continuous Machine Learning represents a significant shift in how we develop, deploy, and maintain AI models. By embracing CML, organizations can ensure their models remain accurate, relevant, and efficient in a rapidly changing data landscape. As this paradigm gains traction, it will undoubtedly drive the next wave of innovation in AI, transforming industries and enhancing our ability to make data-driven decisions. Implementing Continuous Machine Learning requires commitment and collaboration, but the rewards are substantial. By staying on the cutting edge of ML practices, businesses and researchers can unlock the full potential of their AI initiatives, paving the way for a smarter, more adaptive future.
Consult us for free?
View More